- First Break March 2024 | Opportunistic 4D Using a Regional Non-Repeated 4D Monitor, an Ærfugl Case Study
This case study from the Ærfugl field in the Norwegian Sea, shows that MultiClient datasets can be used opportunistically for a better understanding of reservoir production in a time-lapse project, especially when major production variabilities have been observed at various wells in the field since the last dedicated 4D monitoring survey was acquired.
In the example showcased here, it was possible to retrieve an interpretable 4D signal even though the MultiClient acquisition was only repeating the sail-line azimuth direction. Customized processes were used, and special care had to be taken regarding the handling of inherent discrepancies in the 4D signal-to-noise. Continuous communication between geophysicists and 4D interpreters is key for validating every processing/imaging step.
As expected, combining both MultiClient and dedicated 4D surveys for a 4D project constitutes a technical challenge associated with uncertainties related to the non-repeatability of the source and receiver positions. While the legacy 4D surveys (2005-2017) over the Ærfugl field were optimized in terms of dual source and streamer geometry repeatability, the ‘4D opportunistic’ MultiClient data from 2022 was acquired with a larger sail-line spacing and wide-tow triple sources to maximize acquisition efficiency (see the survey design configuration below). The sail-line azimuth of the MultiClient dataset was the only navigation feature in common with the 4D baseline surveys and no effort was made to match the shooting direction (shown in the second figure below).
Acquisition survey design scheme for the three surveys.
Source and receiver positions. 2005 (black) vs 2017 (red) repeated dual sources (A), dual sources 2005 (black) vs wide-towed triple sources 2022 (purple), non-repeated (B). (C) and (D) display the repeatability of the streamer geometry. The 2022
MultiClient dataset was acquired mostly in the opposite direction.
Geometrical Repeatability Assessment
A repeatability study was carried out using only shot/receiver coordinates. Different 4D binning strategies were evaluated by analyzing attribute maps. The most useful attributes for understanding geometrical differences were the sum of the source and receiver distances (dSdR) and the fold of coverage.
Despite having the same acquisition azimuth for the three surveys involved, the 2022 survey was unfortunately acquired in the opposite direction for most of the area. This made it necessary for all 4D binning work to utilize the reciprocity of sources and receivers. Although the dSdR values were unconventionally high, up to 500 m for near offsets, it was decided to proceed with the processing of a small area through a full 4D sequence.
4D Processing Key Steps
Several case studies of opportunistic 4D projects using a non-repeated dataset can be found in the literature. These were mostly conducted for reservoirs with a strong 4D signal. In the Ærfugl case, the 4D signal was expected to be quite weak, with small amplitude differences and time-shifts of less than 1 ms. For this opportunistic 4D project, specific care had to be taken during the processes of signal calibration, receiver deghosting, denoising, and demultiplex (see angle stack below). All three datasets were reprocessed jointly from raw data to ensure the best repeatability in terms of processing sequences and algorithms.
3D QC full angle stack. Monitor dataset 2022. Before demultiple (top), after demultiple (bottom). An arrow indicates a reservoir reflection.
4D Observations and Interpretation
Attribute extractions at target horizons are useful in 4D interpretation as they exhibit higher signal-to-noise ratios and can track time-lapse seismic changes. Normalized Root-Mean-Square (NRMS) of the seismic difference between baseline and monitor surveys is commonly used to quantify repeatability quality. 4D reservoir changes were assessed using the sum of positive amplitudes (SPA) and the sum of negative amplitudes (SNA) of the 4D difference to track hardening and softening respectively.
The final results from the repeated 2005 versus 2017 datasets gave NRMS values in the target interval of 10%. NRMS values for non-repeated pairs, involving the 2022 dataset, were 13% for 2017 versus 2022 and 15% for 2005 versus 2022. Overall, the level of 4D noise is higher on the non-repeated datasets, which was expected as repeating the source/receiver coordinates and source set-up will always give a lower 4D noise level. The level of 4D noise on the non-repeated datasets was, however, low enough to reveal an interpretable 4D signal.
The final 4D products show both hardening and softening effects (respectively blue and red in the figure below). The hardening is interpreted as a combination of pressure decline and water replacing gas. This agrees with the modeled 4D response (labeled A in the figure below), where a weak hardening effect, due to pressure decline, can be seen at the well level, combined with a stronger hardening down-flank, related to water movement. The softening effects are interpreted as an increase in gas saturation below the initial gas-water contact. The increase in gas saturation could come from gas out of solution in the aquifer or local gas expansion as the pressure in the reservoir is decreasing. The 4D effects are close to the noise level in the data, but the availability of multiple monitors enables the interpreter to link the 4D effects between the different production periods and build confidence in the visible signal.
Crossline section, A) the feasibility 4D model 2022-2005 B) 2005 3D stack C) 4D difference 2017-2005 D) 4D difference 2022-2017 E) 4D difference 2022-2005.
MultiClient Datasets Prove Useful for Better Understanding of Reservoir Production
As expected 4D noise levels are increasing significantly when non-repeated acquisitions are used. However, this study has shown that opportunistic 4D datasets can be beneficial if the acquisition time-lapse corresponds to critical reservoir production changes. The success of any opportunistic 4D imaging depends on the use of customized processes and careful testing and QC. In the Ærfugl example discussed here, several factors have contributed to the success of the project.
Firstly, it has proven essential that communication between the imaging contractor and the field operator takes place continuously during the project. Also, 4D objectives and well-production information have to be shared. Continued dialogue between both parties about the interpretation of the 4D results has enabled the tailoring of the 4D processes throughout the project.
Secondly, multi-vintage studies allow us to link the 4D effects between the different production periods. Considering that the noise has no spatial consistency in the time-lapse domain, it is simpler to understand and validate complementary 4D effects using the relationship between survey pairs.
In summary, adding more datasets is helping the better understanding of reservoir production in a time-lapse project even if the extra dataset is not acquired in an ideal 4D-friendly way. However, opportunistic 4D surveys are certainly no substitute for dedicated repeat 4D surveys.
Although the Ærfugl example discussed here specifically focuses on the value opportunistic 4D can bring to an improved understanding of reservoir production it is also worth highlighting that MultiClient data can also be used opportunistically in a 4D ILX (infrastructure lead exploration) setting, where one hopes to detect 4D effects related to gas coming out of solution due to induced pressure changes via production in nearby field.
This paper was published in the March 2024 issue of First Break. The authors are; Didier Lecerf, Stepan Marinets, Sylvain de Pierrepont, Vladimir Zhelanov, Anastasiya Tantsereva, Julien Oukili, Ross Milne (AkerBP) and Anne Stav (AkerBP).

Contact Didier Lecerf
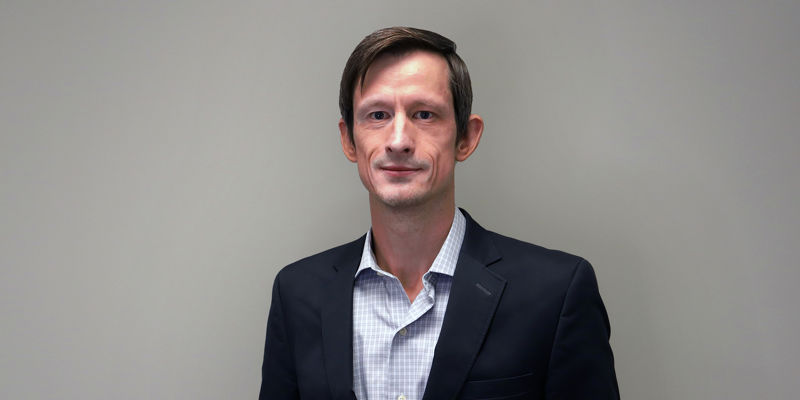